21 Adding AI to Your Course: Diversity, Equity, and Inclusion (DEI) Research Opportunities
Rick Kates, Ph.D.
Background
Artificial Intelligence (AI) and Machine Learning (ML) in health care can improve patient outcomes and influence population health related to Diversity, Equity, and Inclusion (DEI). AI can be applied to larger datasets to be more precise when focusing on public health issues by sorting out specific groups in a population (Newton et al., 2018). Another public health benefit of ML is it can analyze patterns of data for surveillance and disease detection, such as foodborne illness, using Google search and phone GPS data (Sadilek et al., 2018). There is a balance between the benefits and the risks of AI and ML.
There are concerns about biases emerging and recommendations to consider inclusivity to avoid health care disparities in different health care specialties (Adamson & Smith, 2018). The speed at which AI and ML health care innovations are emerging requires a shift in teaching and reviewing of data. Medical education is anticipating changes with the implementation of AI, where physicians will need to understand their patient’s relationship with AI and have a greater awareness of social justice issues related to AI (Rampton et al., 2020).There is a balance between the benefits and the risks of AI and ML.
Diversity, equity, and inclusion (DEI) are core values in almost every mission statement in higher education. Higher education and academic conferences are increasingly recognizing that DEI frameworks are fundamental concepts in curriculums, workshops, and events to the point that entire conferences are dedicated to DEI. UF has several conferences that address DEI; some examples include the UF MBA Diversity & Inclusion Conference, Diversity Week at the College of Medicine, and PHHP Diversity, Equity and Inclusion Week. These types of events offer an opportunity to address diversity, equity, and inclusion related to AI and ML and a way to introduce students to research and provide them with a line for their CV or resume to enhance their career opportunities.
In addition, combining an existing AI or ML project with a DEI can help faculty efficiently address DEI and meet some of the core values of the college or university. However, meeting and delivering on these values can be challenging because often, faculty are apprehensive about teaching DEI concepts, fearing they will make mistakes managing difficult conversations such as racism (Lupton & O’Sullivan, 2020; O et al., 2019).
…combining an existing AI or ML project with a DEI can help faculty efficiently address DEI and meet some of the core values of the college or university. This Adding AI to Your Course lesson proposes introducing DEI events in parallel with an existing AI project or creating a new AI project specific to a faculty member’s discipline. The other recommendation is to make the abstract or poster submission optional. Providing a choice and letting students take ownership in the education has been shown to positively influence their learning (Conley & French, 2014; Pawson & Poskitt, 2019).
Additional recommendations and examples will be provided later in this document. Still, faculty should be prepared to discuss the potential for using secondary data and point students towards government agencies that share datasets to get the data in their hands. Secondary data plays an essential role in public health and other disciplines, and having the ability to explore actual data can increase student learning and engagement (Boslaugh, 2007; Spurlock & Spurlock, 2020). There is an additional opportunity to raise awareness of the potential of technology to address the structural, social, environmental, economic, or political factors that contribute to inequities. For this Adding AI to Your Course lesson, the aim is to identify if students will take additional ownership of their education and career development if authentic learning opportunities, instructional modeling, and research instruction are provided in parallel with existing assignments.
Example and Recommendations
Initial Assignment and Lesson Modeling
Motivational Announcement and Additional Secondary Data Part 1
Motivational Announcement and Additional Secondary Data Part 2
Abstract from This Lesson
Diversity, Equity, and Inclusion (DEI) Research Opportunities: Adding Complementary Learning to Emerging Health Technology/Artificial Intelligence Lessons
Rachel Bennett, Brandi Caughorn, Noah Hammarlund, Frederick R. Kates
Introducing DEI in parallel with an existing lesson, an Emerging Health Technology/AI Project, can promote students’ self-directed education and identify opportunities to collaborate on DEI initiatives in their respective areas of interest. There is an additional opportunity to raise awareness of the potential of technology to address the structural, social, environmental, economic, or political factors that contribute to inequities.
Higher education and academic conferences are increasingly recognizing that DEI are fundamental concepts in curriculums, workshops, and events to the point that entire conferences are dedicated to DEI. Can providing additional research tools and promoting the opportunity to submit an abstract motivate students to take increased ownership of their education?
Lessons were modified to apply a DEI lens to emerging healthcare technology by identifying disparities via the introduction to secondary data sets and the opportunity to submit an abstract to the DEI conference. Students were also encouraged to think critically in their analysis of the technologies available as health disparities research has shown that often technology reflects the damaging gaps in current structures and in some cases make DEI issues worse.
Of the 21 three-person research teams, 19% submitted an abstract to PHHP’s DEI Conference. One team indicated ambitions to develop an AI-based risk score and their abstract submission has been a starting point for their undergraduate research. The integration of DEI into STEM curriculum, paired with the potential to present research projects at DEI Week 2022 gives students experience with data analysis while familiarizing them with related issues in DEI.
Student Examples
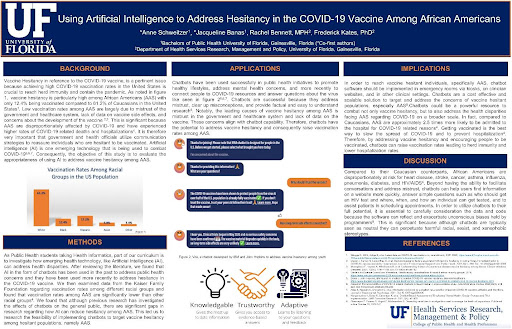
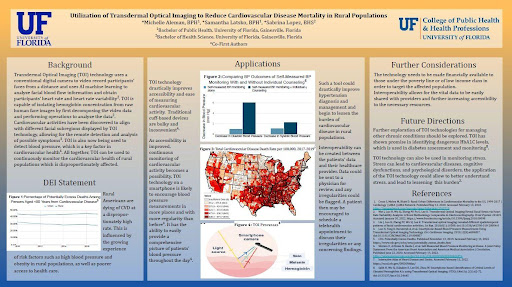

References
- Adamson, A. S., & Smith, A. (2018). Machine Learning and Health Care Disparities in Dermatology. JAMA Dermatology, 154(11), 1247–1248. https://doi.org/10.1001/jamadermatol.2018.2348
- Boslaugh, S. (2007). Secondary Data Sources for Public Health: A Practical Guide. Cambridge University Press.
- Lupton, K. L., & O’Sullivan, P. S. (2020). How Medical Educators Can Foster Equity and Inclusion in Their Teaching: A Faculty Development Workshop Series. Academic Medicine, 95(12S), S71. https://doi.org/10.1001/jamadermatol.2018.2348
- Newton, J., Ekpe, M., & Bradley, P. (2018). Predictive prevention and the drive for precision public health—UK Health Security Agency. https://ukhsa.blog.gov.uk/2018/11/20/predictive-prevention-and-the-drive-for-precision-public-health/
- O,’ Connor M. Rebecca, Barrington, W. E., Buchanan, D. T., Bustillos, D., Eagen, Torkko Meghan, Kalkbrenner, A., Laing, S. S., Reding, K. W., & de, C. A. B. (2019). Short-Term Outcomes of a Diversity, Equity, and Inclusion Institute for Nursing Faculty. Journal of Nursing Education, 58(11), 633–640. https://doi.org/10.3928/01484834-20191021-04
- Rampton, V., Mittelman, M., & Goldhahn, J. (2020). Implications of artificial intelligence for medical education. The Lancet Digital Health, 2(3), e111–e112. https://doi.org/10.1016/S2589-7500(20)30023-6
- Sadilek, A., Caty, S., DiPrete, L., Mansour, R., Schenk, T., Bergtholdt, M., Jha, A., Ramaswami, P., & Gabrilovich, E. (2018). Machine-learned epidemiology: Real-time detection of foodborne illness at scale. Npj Digital Medicine, 1(1), 1–7. https://doi.org/10.1038/s41746-018-0045-1
- Spurlock, D. R., & Spurlock, D. (2020). Scholarship During a Pandemic: Secondary Data Analysis. Journal of Nursing Education, 59(5), 245–247. https://doi.org/10.3928/01484834-20200422-02
Feedback/Errata